Imagine you’re tasked with boosting data quality for your AI model. You’re at a crossroads, faced with two distinct paths for generating synthetic image data. On one side, there’s Generative AI—fast, adaptable, and capable of producing a wide range of synthetic data with ease. On the other, there’s 3D Simulation Models—focused, precise, and built to replicate the real world in stunning detail. Depending on your needs, one of these approaches is going to be the better fit. But which one should you choose? Let’s break it down.
Generative AI: the creative engine
Generative AI is like a highly talented, if slightly unpredictable, assistant. You feed it examples of images, text, video, or sound, and it can create new, similar content. Think GANs (Generative Adversarial Networks), transformers, and diffusion models. This type of AI isn’t just recreating what it’s seen—it’s making something new based on patterns it’s learned. That’s why it’s been such a hit in fields like content creation and entertainment.
the market is moving fast
Generative AI is no niche technology. By 2022, it had already reached a value of $10.63 billion, and it’s expected to grow by over 34% annually through the next decade. A big reason for this is its versatility—Generative AI can do everything from generating synthetic images for testing models, to creating entirely new videos from text prompts (looking at you, DALL-E). In marketing, generative AI is starting to replace traditional content creation, allowing companies to personalize ads and graphics at a scale we haven’t seen before.
If you need something like, say, a huge batch of new images for training a facial recognition system, Generative AI can create faces that look real but don’t actually belong to any person. Generative AI models can quickly generate synthetic images at scale. Want thousands of realistic human faces? No problem. For instance, StyleGAN can produce incredibly lifelike portraits that don’t correspond to real people, while DALL-E can create entirely new images from text descriptions.
3D simulation models: The real-world replicators
While Generative AI is all about creativity, 3D Simulation Models are grounded in accuracy. These models are designed to simulate physical environments and conditions, making them perfect for applications where precision and control are key. 3D simulation generates images creating photorealistic 3D models and mimicking real-world lighting, shadows, textures, and physics, allowing you to create synthetic data that mirrors real-world scenarios.
Built for precision
3D Simulation Models offer unparalleled control over the environment. For example, you can simulate different lighting conditions, weather patterns, or camera angles in a virtual setting. This makes simulation ideal for industries like autonomous driving, robotics, and medical imaging, where the image data needs to be as close to reality as possible. A self-driving car, for instance, can be trained on millions of simulated road scenarios, testing how it reacts to pedestrians, rain, or night driving without the risks of real-world testing.
Market impact
The synthetic data generation market, including 3D simulation-based data, is seeing impressive growth across a range of industries. In 2023, the global market was valued at approximately $218.4 million, with projections estimating it will grow at a 35.3% CAGR through to 2030. What’s fueling this rapid expansion? It’s the increasing demand for high-quality, diverse datasets that power AI and machine learning applications, particularly in fields where real-world data collection can be expensive, risky, and or impossible. Synthetic data—especially the kind generated through detailed 3D simulations—offers a practical, cost-effective alternative to traditional data collection methods
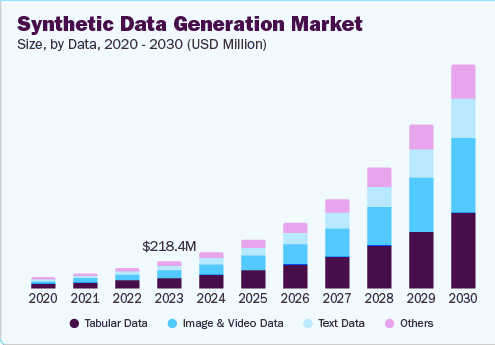
boosting data quality: generative ai vs. 3d simulation models
Generative AI: Perfect for projects where creativity and you don’t need control over specific details. Generative AI can produce a wide array of images from minimal input, making it ideal for industries like marketing, entertainment, and even deepfake creation. It’s fast and scalable, particularly suited for generating large datasets in a short amount of time.
3D Simulation Models: If realism and accuracy are critical, 3D simulations are the way to go. They are indispensable for industries like autonomous vehicles, medical imaging, and robotics, where precise, real-world conditions must be replicated to ensure the AI behaves appropriately in real-world scenarios.
data quality and control
Generative AI: Excels at creating diverse images, but it can sometimes produce unrealistic outputs or artifacts, especially if the training data is limited or poorly curated. While it’s incredibly powerful for generating a wide range of synthetic data, it lacks fine-grained control over specific environmental variables.
3D Simulation Models: These models provide absolute control over every element in the environment. You can tweak lighting, object placement, and other physical interactions with exact precision. This makes it ideal for training AI systems that require a detailed understanding of how objects behave in the real world.
scalability and cost
Generative AI: Once trained, Generative AI can churn out data quickly and at relatively low costs. However, the initial training phase can be resource-intensive, requiring large datasets and considerable computational power. It’s excellent for applications where you need a vast amount of varied data fast.
3D Simulation Models: Creating 3D simulations can be more expensive and time-consuming upfront, as it requires building detailed virtual environments. However, once built, these simulations are reusable and can be adapted for countless scenarios, providing long-term value in industries where safety and precision are critical.
the bottom line
If your goal is to generate synthetic image data for creative industries—whether that’s making marketing visuals, testing facial recognition systems, or creating art—Generative AI is your go-to tool. It’s quick, scalable, and perfect for producing large volumes of diverse images.
But, if your project requires real-world accuracy—such as testing an autonomous vehicle in different weather conditions, simulating how a medical device interacts with human tissue, or building AI models that interact with physical environments—3D Simulation Models are the better fit. They provide the precision and control needed to replicate real-world scenarios, ensuring your AI is trained in a safe, predictable environment.
In the end, both approaches are powerful, but they excel in very different contexts. The choice between Generative AI and 3D Simulation Models comes down to what matters most for your project: creativity and diversity or accuracy and control.
Discover more about synthetic data in our article “The 5 Key Questions About Synthetic Data Every Data Scientist Should Know”.