In this article, we tackle the 5 key questions about synthetic data that every data scientist must understand to stay ahead in the rapidly evolving world of AI. From its creation process to its real-world applications, uncover the answers that demystify this cutting-edge technology and explore why synthetic data is a game-changer for AI. Dive in and unlock the potential of synthetic data today!
1. How does photorealistic 3D simulation enhance the quality of synthetic data for computer vision?
Photorealistic 3D simulation—or digital twinning— is a groundbreaking advancement that transforms how synthetic data is generated for computer vision applications.
By replicating real-world environments and objects in stunning detail, this technology enables the creation of high-quality synthetic data specifically designed for advanced computer vision training.
With fine-grained control over scene parameters and object attributes, developers can manipulate the simulation to create any number of real-world scenarios, helping models learn in a way that’s far more precise and effective than traditional methods.
The beauty of 3D digital twinning is how it integrates real-world physical constraints and domain-specific knowledge into these simulations, making them not only visually indistinguishable from reality but also behaviorally accurate.
This results in the generation of diverse, high-fidelity data sets that simulate real-world data distributions, offering a more controlled yet dynamic environment for training. This ensures that models are exposed to a wide range of conditions, improving their ability to generalize and perform in unpredictable real-world situations.
In essence, photorealistic simulation enhances the quality of synthetic data by creating environments that closely mimic reality, producing diversely trained, more adaptable computer vision models. With this technology, you’re not just generating synthetic data—you’re simulating your world.
2. What techniques are employed to minimize the domain gap between synthetic and real data?
Minimizing the domain gap between synthetic and real data is crucial to ensuring models trained in virtual environments perform seamlessly in the real world. This can be achieved through several advanced techniques.
Hyper-realistic rendering is the first line of defense, recreating real-world lighting and material properties so convincingly that the synthetic data becomes almost indistinguishable from the real thing.
Next, we turn to domain randomization, which introduces controlled randomness into synthetic scenes—adjusting everything from lighting to object positions—to teach models how to handle a variety of real-world conditions.
Another essential technique is fine-tuning environmental parameters to reflect the specific settings where the model will be deployed. Whether the model is navigating a warehouse or a bustling city street, these adjustments help it prepare for the exact challenges it will face.
Blending synthetic and real data into hybrid datasets leverages the strengths of both, providing models with the flexibility and depth required to excel in real-world scenarios.
These techniques work together to bridge the gap between synthetic training and real-world performance, ensuring that models can hit the ground running with minimal additional tuning.
3. How can synthetic data generation accelerate AI development workflows?
Integrating synthetic data into AI development pipelines delivers some serious efficiency boosts, streamlining the process in ways that traditional methods just can’t match.
First off, synthetic data allows for the automated creation of large-scale, diverse datasets, eliminating the bottleneck of having to collect real-world data. Even better, it comes with perfect ground truth annotations, so the painstaking process of manual labeling is no longer a concern. This frees up valuable time and resources for more important tasks.
Another huge advantage is the ability to rapidly iterate on scenario generation and data augmentation. Need a new training scenario? You can generate it with just a few tweaks, speeding up the process and allowing for more frequent model training cycles.
Synthetic data also makes it easy to simulate rare events and edge cases that are difficult to capture in real life but essential for robust model training.
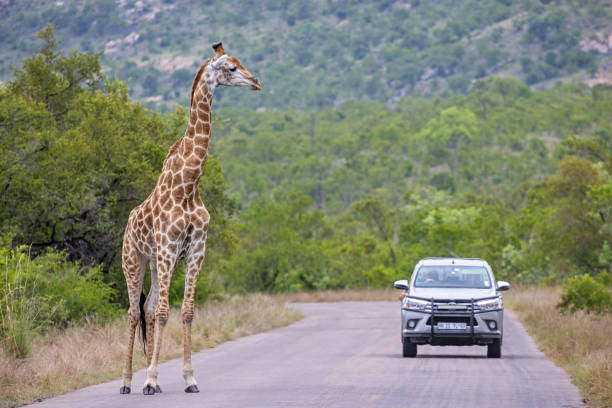
All these benefits significantly reduce development time and costs, enabling teams to shift their focus toward what really matters—improving model architecture and performance.
4. What key factors should be considered when tailoring synthetic data generation to meet specific industry needs?
Adapting synthetic data generation to meet the needs of specific industries requires a tailored approach that goes beyond a one-size-fits-all solution.
First, it’s essential to incorporate domain-specific objects, environments, and scenarios. Whether it’s simulating traffic for autonomous vehicles or medical tools for surgical robots, the synthetic data needs to reflect the real-world context the AI model will operate in. Next, adhering to industry standards and regulations is a must. In fields like healthcare or aerospace, compliance with strict regulations ensures that the data generated is not only accurate but also legally usable.
Another key factor is simulating industry-specific lighting conditions and sensor characteristics. For example, the lighting in a factory setting or the sensor output from a medical imaging device must be captured accurately for models to perform reliably in the field.
Balancing photorealism with the need for diversity and edge case coverage is also crucial—while it’s important to create lifelike data, you also need to account for unusual or rare scenarios that the model may encounter.
5. How is the quality and utility of synthetic datasets evaluated?
Evaluating the quality of synthetic datasets is like giving them a real-world test drive to see how they perform. It’s not enough to just generate the data—you need to know it’s doing its job.
First, we use quantitative metrics to compare how closely the synthetic data matches real-world data distributions. If the data closely aligns, you’re off to a good start. This helps ensure that the models trained on synthetic data will behave as expected when exposed to real-world inputs.
Another important factor is analyzing the dataset’s diversity and its coverage of the target domain. A good synthetic dataset won’t just cover the basics—it will include a broad spectrum of scenarios, including edge cases, to fully prepare the model for any situation.
But the real proof comes when you plug the synthetic data into your benchmarked model. If the model’s performance improves, you know you’ve struck synthetic gold. After all, there’s no better test than seeing how the data impacts real results.
With these strategies, you can confidently gauge whether your synthetic dataset is meeting the high standards needed for top-tier AI training and deployment.
Not sure what type of synthetic data generation is right for you? Check out our article “Boosting Data Quality: Simulation-Based vs. Generative AI Synthetic Data Generation.”